FAQ: How the Iris.ai machine processes thousands of scientific documents
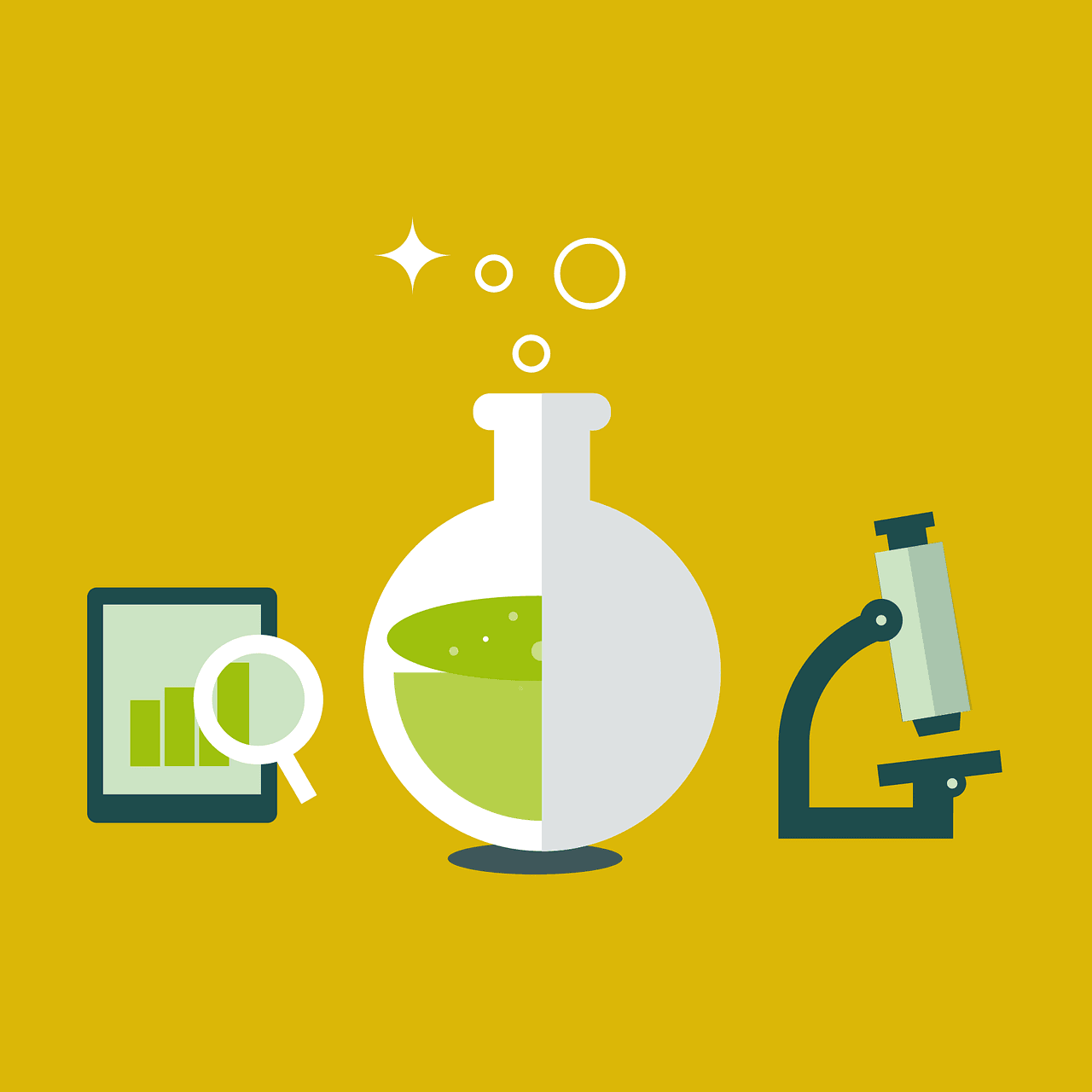
We get loads of questions from our customers who are very curious about what we do, but don’t quite understand how the Iris.ai machine processes thousands of scientific documents. That’s why we’ve collated some of these questions in an old fashioned frequently asked questions.
If you have any other questions or would like a demo, we’re only one click away.
How do I train the machine in my research domain?
Training the machine on your specific research domain includes three steps:
A good description of the company’s area(s) of operation
Simple output data layout (only for data extraction)
Feedback loop with you, the domain expert, for validation of initial results
Do I need to retrain the system for every product or disease I want to do reviews in?
No, as long as you work in the overall same field, it will be sufficient to train the system on that topic. However, if you are part of a major conglomerate that works across a large and very broad number of topics, it might be beneficial to have a handful of trained models in different areas. Most smaller providers or departments will not have this need.
When discovering relevant literature or extracting data, how does the AI machine establish links between scientific documents, specific entities and my research context?
This is a big question, but we’ll try to break it down. The Iris.ai core machine learns your research domain and context during the training phase (see question on training the machine). When fully trained, the machine is able to identify relevant literature and extract relevant data from your documents by identifying the most meaning-bearing words in your documents.
The machine then enriches these words with contextual synonyms and topic words to build a contextual ‘fingerprint’ which is matched with the content collection or database of scientific text the tool is connected to.
This allows you, the user, to link a self-written problem statement or a document against all available research papers, patents and other scientific text sources to find the most relevant knowledge. Using the same “fingerprint”, the machine identifies various values and entities in the documents, which it extracts neatly.
If you want to pop the hood of our AI engine, check out our peer-reviewed Open Access research.
How does the tool know what relevant data to extract?
One of the configuration parameters for the tool is what we call the output data layout. As a client, you specify the data you’d like to extract to this layout.
Can Iris.ai extract data from text as well as tables?
Yes, Iris.ai extracts entities and data from both text and tables. Moreover, when there are data points in the text, which are related to data points in tables, the machine makes those connections in the extraction output.
How about image-based PDFs? Can the machine process that?
Yes, the machine can process images-based PDFs, but this requires evaluation and incorporation of an external OCR tool (optical character recognition). That’s not a problem for us to do.
What document formats does the machine accept? (If I’d like to upload a bank of scientific documents for the machine to review.)
Any machine-readable PDF with text and/or tables of scientific and technical text can be inputted. However, the further away from patent formatting, the higher the chance of requiring retraining (see question on training the machine).
What’s the output format for data extraction?
With the help from us at Iris.ai, you’ll set up the desired output data layout for the data extraction, based on how you want the output to look like (e.g. headers).
How can I export the data?
When you have extracted all the data from your documents, you can export the data to CSV or connect your system with our APIs.
What does it look like for the end user?
Better to look for yourself! Here’s a short demo of our extraction tools for material science.