FAQ about the Researcher Workspace
We get loads of questions from our customers who are very curious about our new tool – the Researcher Workspace. That’s why we’ve collected some of these questions in an old-fashioned frequently asked questions.
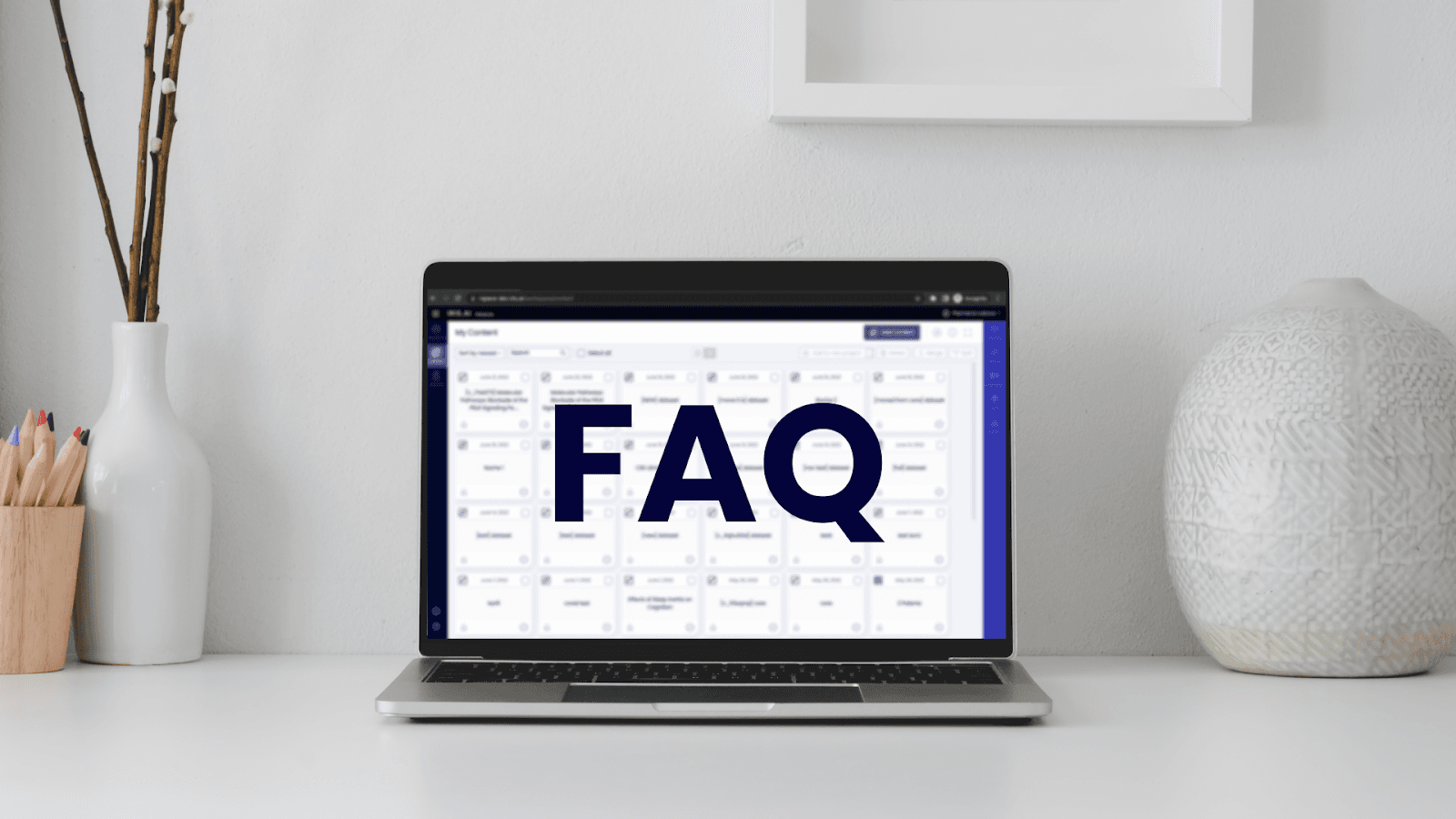
If you have any other questions or would like a demo, we’re only one click away.
How is the Researcher Workspace different from other tools available online?
The Researcher Workspace is an AI-based research platform. What differentiates it from other tools available online is the all-in-one approach. The researcher or a science enthusiast can start an interdisciplinary search, find broad lists of articles, filter the most relevant ones, summarize their findings and lastly extract the data points – all of that using one platform! Moreover, the platform is based on artificial intelligence models, which means that the machine really understands what you are looking for and it is able to find scientific articles that other keyword-based search engines are not.
How should I get started with the tools?
Using completely new tools might be an overwhelming experience and a challenging process – we understand that! Therefore, when your business acquires the license your researchers will receive several training sessions, help materials with all steps and FAQs as well as video tutorials. For the individual users – don’t think we have forgotten about you, stay tuned for the official release in the fall.
After adding the content, the Researcher Workspace tools are interchangeable – you can combine them in any way you want. For example, you can start with an open repository dataset and then Explore the documents within by using your problem statement or link to relevant articles or you can upload your own dataset and use the smart filters to narrow down the list. Every research process is different and you can use the tools however it makes the most sense to you.
What types of content do the tools work with?
The tools work with documents of scientific language. This can stretch to trade magazines, popular science, regulatory documentation and internal memos, but the further away from ‘pure’ science we get, the less accurate the tools will get.
The tools work with almost any type of machine readable content:
- PDF
- As a starting point, patents and research papers in PDF formats are the primary source.
- Any PDF document including scientific text and tables of data, so long as the PDF is encoded in any of the (too many) main approaches used.
- Word processing: .txt, word, powerpoint etc
- We can support a range of other formats, so long as they contain obtainable scientific text and/or data.
- Coming soon! Collections: BibTex, RIS and CSV ++
How does the machine know which points to extract?
One of the configuration parameters for the tool is what we call the output data layout. As a client, you specify the data you’d like to extract and together with our team we will create this layout, which then gets implemented and ready to use within the Extract tool.
How do I train the machine in my research domain?
It starts with the client providing some research articles or patents describing his field or specific problem. Then we are using our engine to find thousands of related papers based on each article provided. Then we “inject” found articles into our general model to enhance the relationship of the words that are directly associated to the domain knowledge requested by the client. As a result, our engine becomes more sensitive towards domain-specific terms and their relationship to other words that are already in the model. Feedback loop with you, the domain expert, for validation of initial results.
What is the difference between an abstract and your summary?
There are broadly two approaches to automatic text summarization: extractive and abstractive. Extractive approaches select passages from the source text, then arrange them to form a summary. You might think of these approaches as a highlighter. The summarization that Iris.ai uses is abstractive summarization. This means the tool captures the context of the text and in essence, writes its own summary – it does not just copy-paste parts of the texts together, which is called extractive summaries. The machine-generated summary of Iris.ai thus contains new phrases and sentences that may not appear in the source text.
The Iris.ai’s generated summary takes out the most important parts of the article and unlike the article’s abstract, it is not biased by the authors.
What does it mean that the tool is AI-based?
Artificial Intelligence is a crucial and helpful part of the Researcher Workspace. For example, in the Explore tool, the machine extracts the most meaning-bearing words from your article or problem statement and then enriches them with contextual synonyms and topic words to build a contextual “fingerprint” which is matched with the content collection or database of scientific text the tool is connected to. Using the same “fingerprint” approach in the Extract tool, the machine identifies various values and entities across text, tables, and graphs (!) in the documents, which it extracts neatly. Moreover, the Extract tool has no problems with handling abbreviations, different measuring units, and converting them. The AI is used as well in the Analyze tool to quickly scan the dataset and provide topic and concept lists to filter the articles.
Does the machine also understand other languages?
Currently the machine works only in English, but in specific cases we can implement a translation to other languages. It is worth mentioning that most non-English scientific articles include an English version of the abstracts as well, so our machine successfully works with them too.
Watch our quick 1 minute video to get a quick overview of the Researcher Workspace.
Wanna know more? Get in touch!