Cut through the noise with AI-powered literature search
Something we all can relate to: we’re drowning in knowledge.
What’s becoming more and more obvious is that we need better tools to sort through all the knowledge and pinpoint what’s relevant to us. AI-powered literature search is scientifically proven to be more effective at just that compared keyword-based tools. Read on to learn how and why and see a demo of how it works.
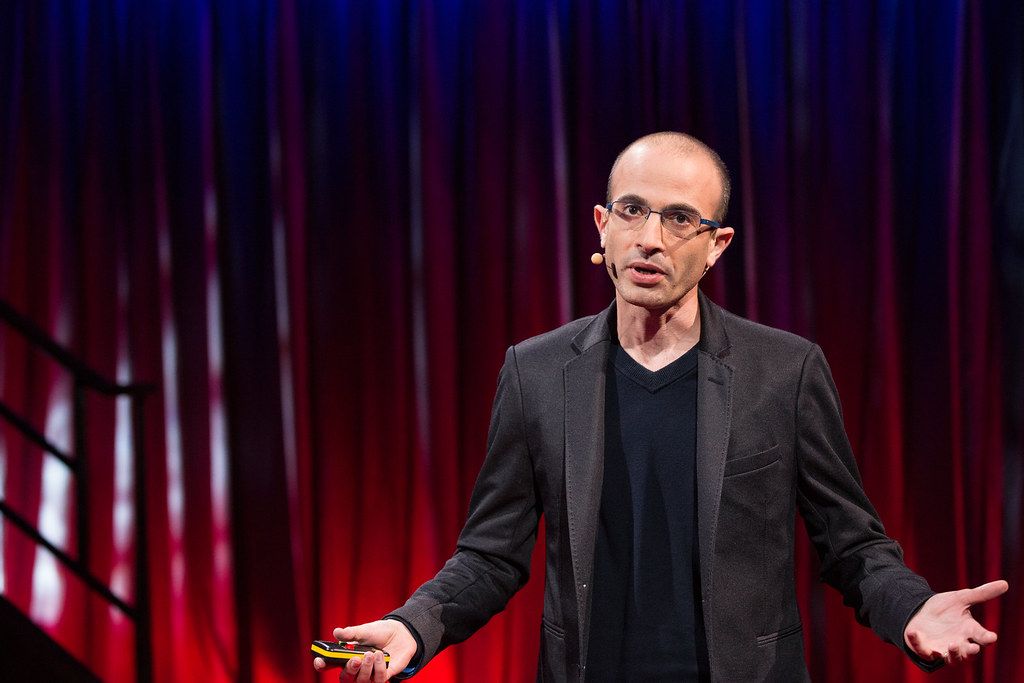
Yuval Noah Harari who’s most famous for his best-selling book Sapiens, wrote in the sequel Homo Deus:
“In ancient times having power meant having access to data. Today having power means knowing what to ignore”.
This is a powerful statement which summarizes the 21st century succinctly in one sentence. We’re drowning in knowledge, and that is particularly true for researchers.
Let’s get the facts straight
The number of published papers and patents grows exponentially. About 2 million papers are published per year, that’s about 5,500 per day. And the growth is growing!
R&D spending has reached a record high level globally, almost US$1.7 trillion. That’s four times the GDP of Norway 🇳🇴🇳🇴🇳🇴🇳🇴 And to no surprise, the biggest spenders are the pharmaceutical and biotechnology industry, which spends on average 15% of its revenue on R&D.
Maybe the most exciting trend is open access and decentralised knowledge networks, which make it easier for everyone to publish and access research papers. For example, scientists publish their articles to a website called arXiv.org before they’re peer-reviewed, as getting a paper peer-reviewed takes 9–18 months — and in today’s world that means the knowledge will be out of date.
In other words, we can see that knowledge is increasing exponentially, and that Yuval Harrari is right in saying that power means having the tools to eliminate the noise and being able to focus on the information that matters. And that’s true for literature search too.
AI-powered literature search — scientifically proven
Researchers like science, which is why we decided to scientifically prove the effectiveness of AI powered literature search. We wanted to assess the usefulness of various tools in conducting research mapping exercises, and directly compared Iris.ai with the likes of Google Scholar. We want to emphasise that the method is peer-reviewed and published.
AI-powered literature search vs. keyword-based search
We headed over to Gothenburg in Sweden to team up with RISE, a leading institute focused on composite materials. At RISE, we set up two teams consisting of four researchers from interdisciplinary teams who competed against each other to address the research question: “Can we build a reusable rocket exclusively made of composite materials?”.
They had 5 hours to map and categorise scientific papers, articles and reports that were relevant to the research question. One of the teams used Iris.ai to find relevant papers, whilst the other team used Google Scholar.
To assess the results, we had an independent jury of professors at RISE who were experts in the research field of the experiment. They scored the performance of the teams based on three criteria:
- Overview: How well did the team manage to explore the overview of the problem?
- Findings: Are there interesting findings in the results?
- Conclusions: Is the conclusion following the latest trends in research?
Results
After the five hour experiment, the jury gave their evaluation of both teams. The team using Google Scholar got 45% based on the criteria mentioned earlier, and the team using Iris.ai received a score of 95%. In other words, the AI engine boosted one research team’s efficiency remarkably.
How did AI-powered search beat keyword-based search?
- The AI-powered literature search enabled the researchers to find specific relevant papers that the other team didn’t.
- They got a better overview of the field, as they found many more papers that were relevant to the research questions.
- Because the winning team found more relevant papers, they were able to draw better conclusions.
A very funny moment during the experiment which illustrates why the winning team got a score of 95% was when they discovered a paper the jury wasn’t aware of. The newly discovered article baffled the jury so much they had to take a break to check it out. Being professors in the research field of the experiment, they couldn’t wait to see what this paper was about.
Of course, this was a study over a short period of time and with academic researchers, but it translates to other industries. From working with clients in pharmaceuticals, biotech, food and so on, we’ve seen that researchers in any domain who need to find and review scientific documents, find AI-powered tools very helpful for their processes.
How do you get started with AI-powered literature search?
First, you give the machine a good description of the field that your company works in. That can be types of diseases, material, medication or whatever else you’re working on. A couple of hundred words that we use to train the engine so it understands your research domain. The machine takes a couple of days to train itself.
Second, your domain experts verify the initial results. We know AI, but you are the domain expert in your field. That’s why we’ll spend about four hours going through initial results to tweak the machine.
All in all, it’s less than one day in man-power.
Your unique research fingerprint
The way that we’re all unique humans with our own unique fingerprints, the same way your research is unique to the whole world. (Sorry about the cheesy line, but bear with me.)
When you search for literature, you provide the machine with an existing and relevant paper or use your own problem statement. The AI machine will then find the most important words in that paper and identify synonyms and hypernyms. The machine forms your unique research fingerprint, which it matches to documents in the database.
Then the engine scores other literature based on how similar it is to your virtual fingerprint. You can see this score as a percentage, where 100% would be completely similar, or even a duplicate. Your unique search results will contain papers that match your unique fingerprint.
This is often called a content-based recommendation engine. The machine is not showing and recommending what other researchers have read, but only the documents that match the text you uploaded.
This is in stark contrast to traditional search tools where you need to build long and quite complicated strings of keywords to find the relevant scientific documents.
Demo time
To see it for yourself, check our demo. Fast forward to 13:13.
Summary
👉 We’re flooded with irrelevant information and knowledge, which is distracting and exhausting. This is particularly true in research.
👉 Whenever we enter a search query into traditional tools, we get never-ending lists of literature; and it’s an insanely manual job to filter through them to pinpoint the relevant and really useful papers. The researchers who’s getting ahead are training themselves to use tools that can distinguish the irrelevant literature from the relevant one.
👉 AI-powered literature search are trainable in any research domain, and are therefore more able to sort through the noise and find the papers you really need. The good news is that it takes less than a day in man-power to get started.
What’s the best way to get started? You start today. Not next week. Not tomorrow. Today 🙏