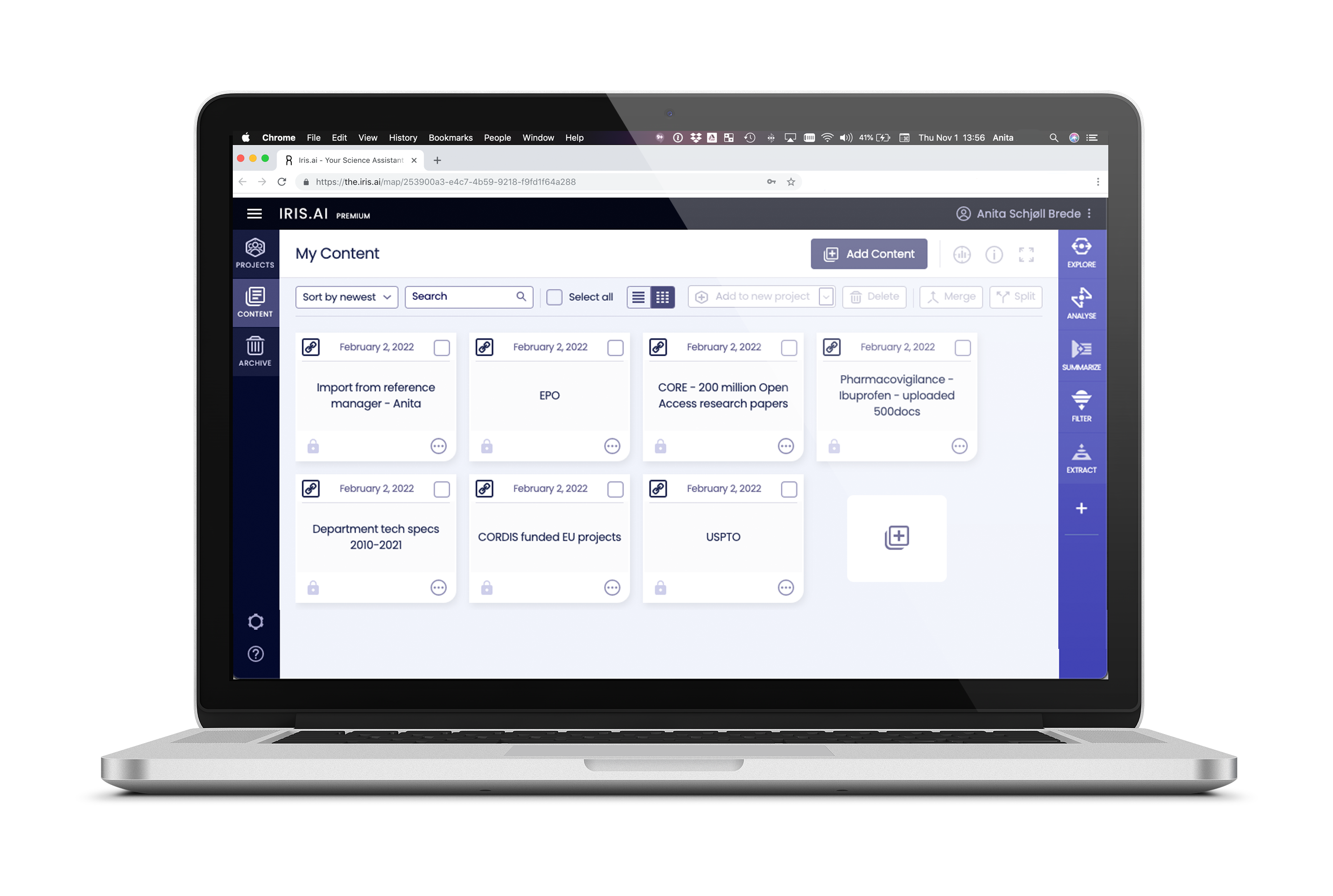
THE RESEARCHER WORKSPACE INCLUDES:

Content based search
Bypass keywords and explore interdisciplinary with a content-based recommendation engine.

Context filtering
Filter down a document set based on criteria you can explain in a sentence, but not put into one keyword.

Data filtering
Filter based on information extracted from your documents like specific entities, data points or data ranges.

Extracting and systematizing data
Automatically extract and systematize any key data points from text and tables into a table layout of your own design.

Document set analysis
Analyze a large set of documents to form an overview of the content – and decide what to include and exclude.

Summarization
Ask the machine to summarize single or multiple documents to get a quick overview, or kickstart your writing.

Chatting with dataset
Interact with your datasets to distill meaningful insights and summarize findings.

New challenges?
Have a specific, scientific or medical text related problem you’re not seeing the solution to? Get in touch – we love a challenge!
Upcoming events to learn more
WHY IRIS.AI?
We have spent the last 8 years building an award-winning AI engine for scientific text understanding. Our algorithms for text similarity, tabular data extraction, domain-specific entity representation learning and entity disambiguation and linking measure up to the best in the world. On top of that our machine builds a comprehensive knowledge graph containing all entities and their linkages to allow humans to learn from it, use it and also give feedback to the system. Applying these on scientific and technical text is a complicated challenge few others can achieve.