Why is a horizontal approach to AI the way to go? AI across domains
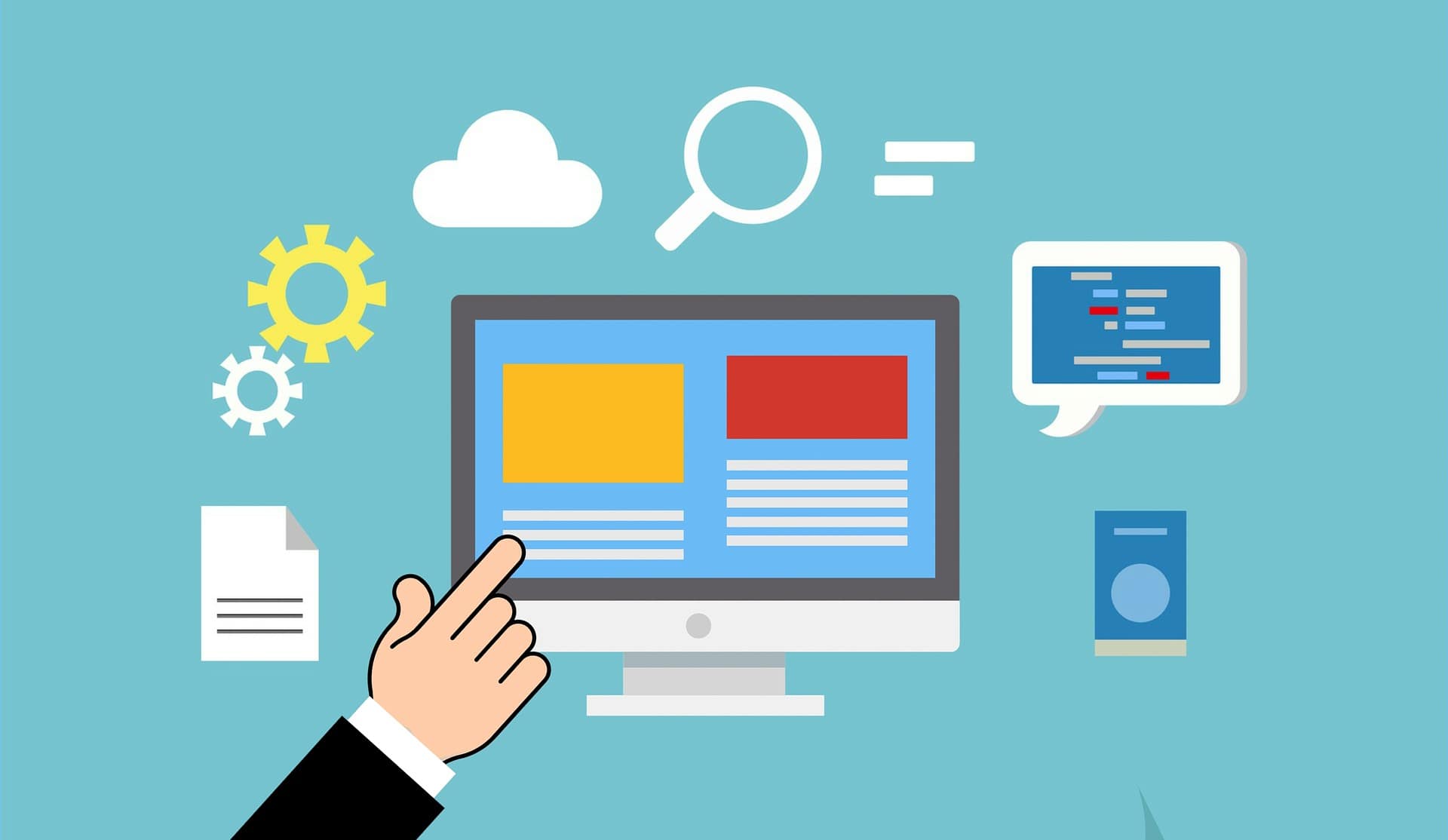
AI can be applied in two different yet equally important and complementary ways: vertically and horizontally. In layman’s terms, vertical AI is applied to a specific problem in a specific industry. It is trained exclusively on that industry-specific data. Vertical AI companies are focused on mastering a single, large use case. Waymo and Vara are two often given examples of such vertical AI companies.
On the other hand, horizontal AI can be applied across different industries and is able to handle multiple tasks. This type of AI is often inspired by our knowledge of how the human brain works while solving an issue. Its goal is to build complex algorithms that can perform diverse tasks from a common core. AI is an automated decision-making system, which continuously learns, adapts, suggests and takes actions automatically. For instance, Apple’s Siri or Amazon’s Alexa are examples of horizontal AI applications.
One way of looking into this distinction is to think of vertical and horizontal AI compared to Isaiah Berlin’s well-known ancient idea that people can be fundamentally distinguished between two types, hedgehogs and foxes. In his groundbreaking essay of the same name, the renowned social theorist and philosopher talks about those who, like the fox, see details in everything they do and those who are great at having one singular vision, like the hedgehog. The message here being that like hedgehogs, vertical AI “relates everything to a single central vision” and horizontal AI “pursues many ends”, like foxes.
To put it in simple words, horizontal AI is to your GP what vertical AI is to a highly specialized surgeon.
Benefits of horizontal AI
Horizontal AI allows companies and startups in particular to quickly adapt modules to fit the needs of the client, so the product’s use can be adapted without having to go back to square one every time. From the company’s perspective, being horizontal gives a lot more flexibility in applying algorithms to a wider market. This is crucial to Iris.ai, considering that our vision is to help researchers around the world to increase their efficiency. Horizontal AI allows us to provide solutions that are both industry-specific and created for a specific task while adaptable to other tasks in different domains or expressed as different use cases.
Taking a horizontal approach has been found to be good for us and for our clients.
The Iris.ai approach
At Iris.ai we are using a horizontal AI strategy. We have a general language model and for different clients we are able to update this model to serve different topics for industry-specific tasks.
So you’re probably wondering, how does the process look like?
It starts with the client providing some research articles or patents describing his field or specific problem. Then we are using our engine to find thousands of related papers based on each article provided. Then we “inject” found articles to our general model to enhance the relationship of the words that are directly associated to the domain knowledge requested by the client. As a result, our engine becomes more sensitive towards domain-specific terms and their relationship to other words that are already in the model. Then, depending on the use case we replace the general model in our tools with a domain-specific model, so that clients can use it to find the articles that are the most relevant to their interests.. As a result, a screwdriver manufacturer using our domain-trained tools will find articles about iron – as a material used in production, not as a generally known chemical element. Training the model doesn’t limit the database – a domain-trained engine has the same databases with articles connected as a general model, but a more narrowly trained model prioritises domain-specific key terms and is tailored to provide more precise and relevant results. Of course, we can also integrate additional databases as per client requests.
For the extraction of data, our clients can choose to provide an ontology – the relationship between key terms and concepts of relevance to their field. For example, a company creating screwdrivers would choose steel, iron etc. as key terms they want to focus on. Then we are using our model to identify other words associated with the key phrases provided. After identifying all key phrases across articles we connect properties mentioned in the text and tables.
Our research team is also developing in-house graph embedding technique, which will be able to link different kinds of keywords in the domain-specific corpus and then transfer them into the general model.
Key Takeaways
Both the horizontal and vertical approaches to AI offer valuable solutions. While horizontal AI focuses on solving a broader-range problem statement, vertical AI focuses on niche. Horizontal AI is particularly helpful to startups such as Iris.ai thanks to the ability to quickly train the general model to service client-specific needs, therefore boosting the scalability of our own internal R&D.