What are AI hallucinations and how we are dealing with factuality issues
In the realm of artificial intelligence, Large Language Models (LLMs) have emerged as groundbreaking tools that showcase the power of Natural Language Processing (NLP). They have the ability to generate human-like text, engage in meaningful conversations, and provide information on a wide range of topics. However, there’s a catch. When it comes to scientific facts, these AI giants often stumble and falter. But why does this happen? In this blog post, we’ll delve into the complexities of hallucinations and factuality issues, their implications, and potential solutions.
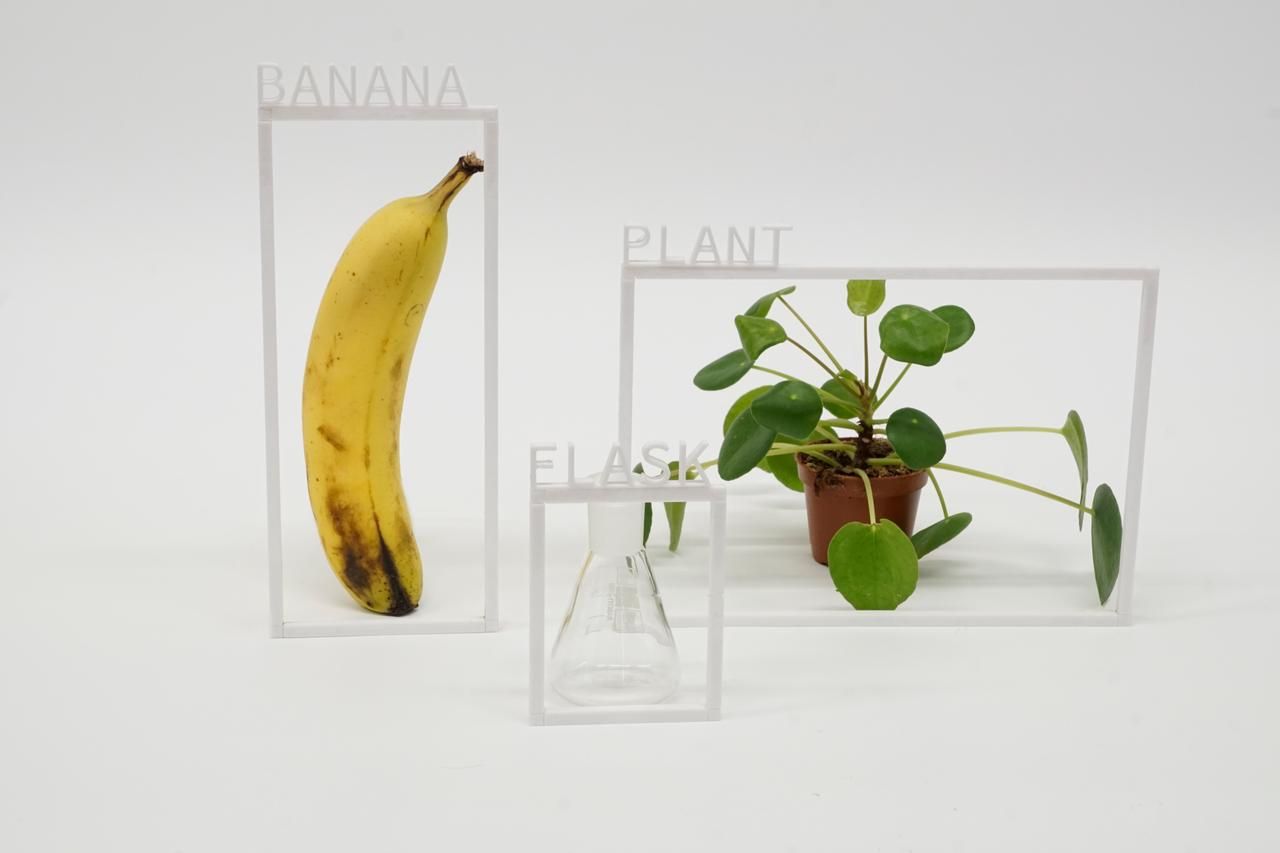
What are AI hallucinations?
AI hallucinations, also known as “adversarial examples” or “AI-generated illusions,” refer to a phenomenon in which artificial intelligence systems, particularly machine learning models, misinterpret or generate seemingly random and incorrect outputs.
Factuality issues with AI refer to instances where AI systems generate or disseminate information that is inaccurate, misleading, or outright false. Hallucinations can undermine decision-making processes, erode trust in AI technologies, and potentially lead to detrimental consequences.
AI hallucinations are more common than you think
Just as with any human creation, AI is susceptible to biases, inaccuracies, and misconceptions. Factuality issues arise when AI systems generate information that, while seemingly credible, is actually incorrect or misleading. This phenomenon is particularly pronounced in natural language processing, where AI-generated text can convincingly emulate authoritative content while lacking factual basis. Therefore it is hard to easily distinguish between facts and fiction. Moreover, the AI won’t tell you it doesn’t know the answer – it will just come up with one!
A real life example of AI hallucinations appeared when Meta demoed Galactica, an LLM designed for science researchers and students. When asked to draft a paper about creating avatars, the model cited a fake paper about the topic from a real author working in a relevant area.
Why does AI have a problem with facts?
The underlying causes for AI’s tendency to produce inaccuracies are:
Generalization
AI models generalize from the training data to answer a wide range of questions. Sometimes, this generalization can lead to inaccuracies or over-generalized statements. Especially when it comes to numerical facts the model usually knows that the next token should be a year – but because of its inherent ability to generalize and paraphrase it might come up with not the right year.
Lack of Ground Truth
During training LLMs don’t usually have a “ground truth” to compare their outputs against. This is different from a supervised learning task where there’s a clear right or wrong answer. The AI tries to predict the next word based on a multibillion parameters function that it learns over the course of training. Thus, it can occasionally produce answers that sound plausible but are incorrect.
Inherent Model Limitations and optimization targets during training
Even state-of-the-art models have their limits. There’s always a trade-off between a model’s size, the amount of data it’s been trained on, its speed, and its accuracy. Even with significant computational power and data, no model can be perfect. One particular aspect is that during training, these models use statistics to predict the next word or phrase that’s most likely to come next, based on their training data. They aren’t necessarily optimized to always provide the most accurate or factual information.
How are we dealing with it
At Iris.ai, we’re acutely aware of the factuality issues that can arise in the world of AI. It’s essential to recognize that these issues are not indicative of AI’s failure but rather a call to action for continual improvement and responsible development.
Addressing factuality concerns requires a multi-pronged approach that intertwines technological innovation, ethical considerations, and ongoing learning:
Robust Training Data
The foundation of any AI system lies in its training data. To mitigate factuality issues, we meticulously curate and vet training data from reliable and diverse sources. Our team ensures that the data used to train our AI models is accurate and comprehensive, minimizing the risk of perpetuating misinformation.
Transparency and explainability
We use ranking mechanisms and all NLP techniques we have at our disposal to explain the given result of our models. For example, our Extract tool comes with an explainability feature. It’s a separate spreadsheet where each of the data points is rated based on the confidence of the machine. If the machine is not sure about extracted data the score is lower. The researcher then can double-check lower scored points therefore preventing any factuality issues.
Using knowledge graphs
At Iris.ai we are working on building knowledge graphs from scientific texts. We are using the generated knowledge graph alongside the different language models to bias the output token generation with the factual information to minimize hallucinations. In other words, the objects and relationships from the knowledge graph are guiding the model towards the factual information instead of using only the probability of the next word generation without any restrictions as in current models. You can read more about knowledge graphs in our blog post: “Tech Deep Dive: Building knowledge graphs”.
Conclusion
AI’s potential to transform industries is immense, but the issue of factuality cannot be overlooked. Factuality issues in AI demand a balanced approach that considers technological advancement, ethical considerations, and social responsibility. At Iris.ai, we’re not just building AI; we’re building AI that respects the facts. Together, we can navigate the complex terrain of AI factuality and shape a future where technology enriches our lives without compromising on accuracy.