How Content Based Recommendation Engine (CBRE) is Reshaping Research Discovery
In the fast-paced world of scientific research, the ability to swiftly access relevant literature can make all the difference. Traditionally, researchers have relied on keyword searches to sift through vast repositories of information. However, as the volume of available literature continues to grow, a new player has emerged—the Content-Based Recommendation Engine (CBRE). In this blog, we’ll explore the benefits and reasoning behind CBRE compared to traditional keyword searches, with a specific focus on the Explore tool.
But what sets CBRE apart from its keyword-based counterparts, such as Google Scholar? Let’s delve into the reasons why CBRE reigns supreme in the world of research exploration.
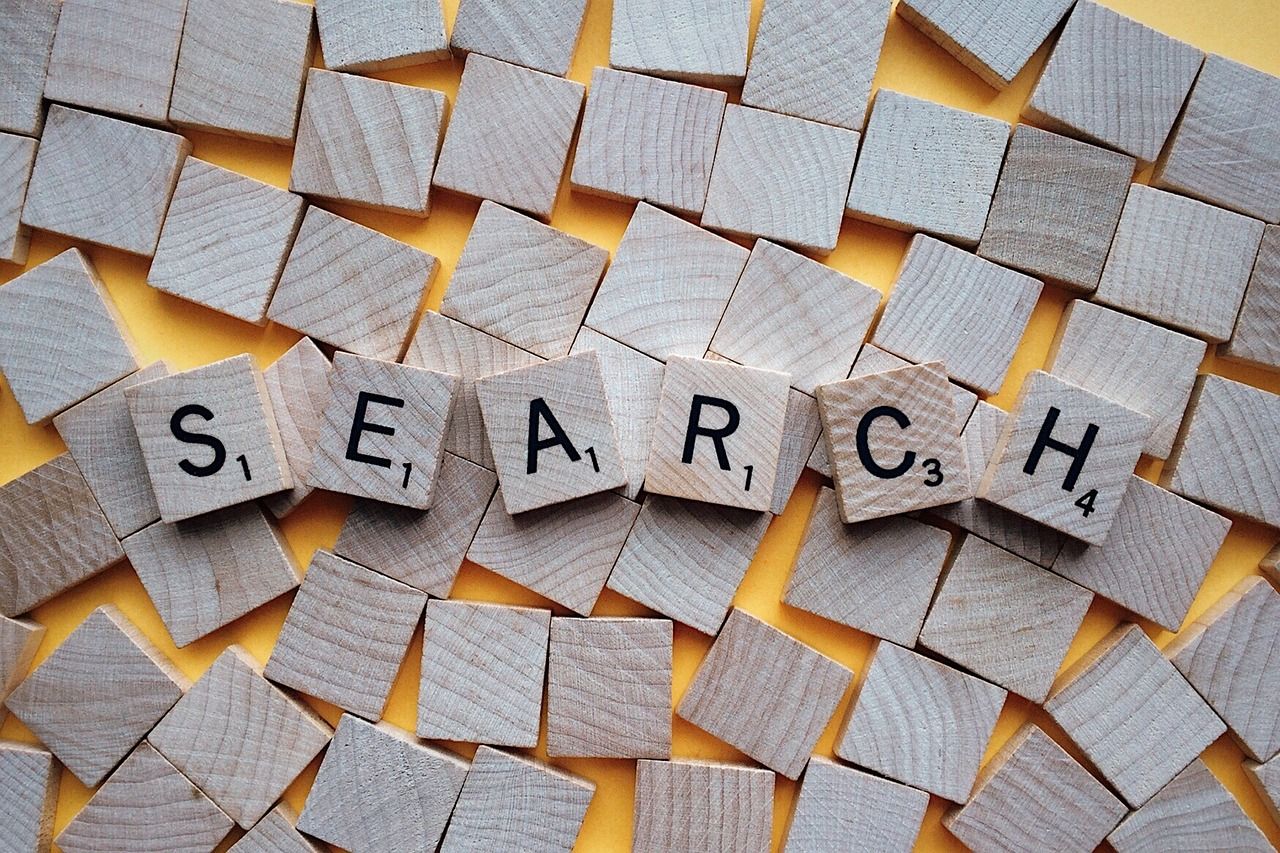
Why Keywords Aren’t Enough?
Keywords have long been the cornerstone of research exploration, offering a straightforward way to find information on specific topics. However, their utility is limited by the user’s ability to articulate their search query effectively. If you know exactly what you’re looking for, keywords can be effective. But what if you’re exploring a new topic, delving into interdisciplinary research, or simply unsure of the vocabulary used in a particular field? This is where the limitations of keyword searches become apparent.
Polysemy and synonymy
Search engines encounter significant challenges when dealing with polysemy, synonymy, and natural language searches. Polysemy refers to words with multiple meanings Similarly, synonymy presents a challenge as different words may convey the same meaning.
Keyword search engines struggle with distinguishing between words that share the same spelling but hold different meanings, such as “hard cider” versus “hard stone.” Stemming issues, singular/plural discrepancies, and variations in verb tenses further compound the problem. Traditional search engines often fail to grasp the nuanced contextual meaning of terms, resulting in irrelevant or inaccurate search results. In essence, keyword searches necessitate users to construct intricate queries, employing Boolean logic to refine their searches—a process fraught with complexities and inefficiencies.
Prioritizing clicks and citations
One common approach employed by traditional search engines is to prioritize search results based on popularity metrics such as clicks or likes. While seemingly intuitive, this methodology has its drawbacks. Popularity-based rankings may lead to a bias towards well-known or frequently cited sources, potentially overlooking lesser-known yet equally valuable contributions to the field. Moreover, reliance solely on popularity metrics fails to account for the relevance or quality of the content, thereby limiting the scope of search results. Similarly, prioritizing search results based on citation numbers is a common practice in traditional search engines. However, this approach often favors established works with a high citation count, potentially overshadowing emerging or niche research. While citations serve as a measure of a paper’s impact within the academic community, they do not necessarily reflect its relevance to a specific research inquiry.
Complex Boolean Keyword Queries
Traditional keyword searches often require users to construct complex queries using Boolean logic, including operators like “and,” “or,” and “near,” as well as parentheses, quotation marks, and more. This process can be time-consuming and prone to errors, detracting from valuable research time. Wouldn’t it be simpler to express your research problem in plain language, allowing the machine to handle context, synonyms, and nuances automatically?
Need for Content Based Recommendation Engines
Natural language searches add another layer of complexity, as users may express queries in diverse ways, requiring search engines to decipher the intent behind the language. These challenges underscore the need for advanced algorithms and techniques, such as content-based recommendation engines, to enhance search accuracy and relevance, especially in the nuanced domain of scientific research.
The Power of CBRE: Introducing the Explore Tool
Enter CBRE—a cutting-edge technology that revolutionizes the way researchers explore and discover literature. At the forefront of this innovation is the Explore tool, a sophisticated platform designed to leverage the power of content-based recommendations. Unlike traditional keyword searches, which rely on specific queries, the Explore tool allows users to input self-written text or a link to a research paper. This flexibility enables users to conduct broader, more nuanced searches that capture the full breadth of relevant literature. Our CBRE leverages advanced word embedding techniques to identify key terms and phrases within documents, forming unique “fingerprints” that encapsulate the essence of each text.
What is fingerprinting?
The method begins by the machine reading either through the paper’s abstract or a self-written problem description. Then the machine identifies the most meaning-bearing words in the text itself, enriches it with contextual synonyms and other words that scientists use within the same context. It also enriches the model by adding hypernyms or topic modeled words. All of that goes into the so-called fingerprint, which is a weighted list of words according to importance.
Once the fingerprint is ready, the next step of the process is to look for similar documents as compared to the input – a.k.a Fingerprint matching. It is based on the Word importance-based similarity of documents (WISDM) metric, which measures the similarity between the Fingerprint and a set of documents revolving around the topic of interest. The end result of the fingerprint matching is a hierarchical list of documents based on the similarity to the input, allowing for a broad definition of every word depicted in the fingerprint.
Visual exploring
By simply providing a self-written text or a link to a research paper, users can generate a map of relevant papers with their relevance scores. The map has a Voronoi diagram structure where the articles are divided into topic clusters. Users then can jump into each of the topics to review the results. This streamlined process accelerates the pace of research and enables researchers to stay ahead of the curve.
Customization and Flexibility
One of the key advantages of the Explore tool is its customization and flexibility. Users can choose which datasets to explore within, adjust publication dates, and select repositories such as PubMed. Additionally, the tool offers a range of filtering options, allowing users to refine their search criteria to suit their specific needs. This level of customization ensures that researchers receive tailored results that align with their research objectives.
Encouraging Serendipitous Discovery
Perhaps most importantly, the Explore tool encourages serendipitous discovery. By presenting users with a diverse array of research papers, including those outside their immediate area of interest, the tool fosters creativity, innovation, and the exploration of new ideas. This deliberate inclusion of seemingly unrelated topics increases the likelihood of stumbling upon unexpected insights, sparking new lines of inquiry, and pushing the boundaries of knowledge.
Conclusion
In conclusion, while keyword searches have long been the go-to method for navigating the research landscape, CBRE offers a superior alternative. Through its flexibility, precision, and ability to encourage serendipitous discovery, the Explore tool is empowering researchers to uncover new insights and drive groundbreaking discoveries. As the volume of available literature continues to grow, embracing innovative tools like CBRE will be essential for staying ahead of the curve in the research.